- Nur online erhältlich
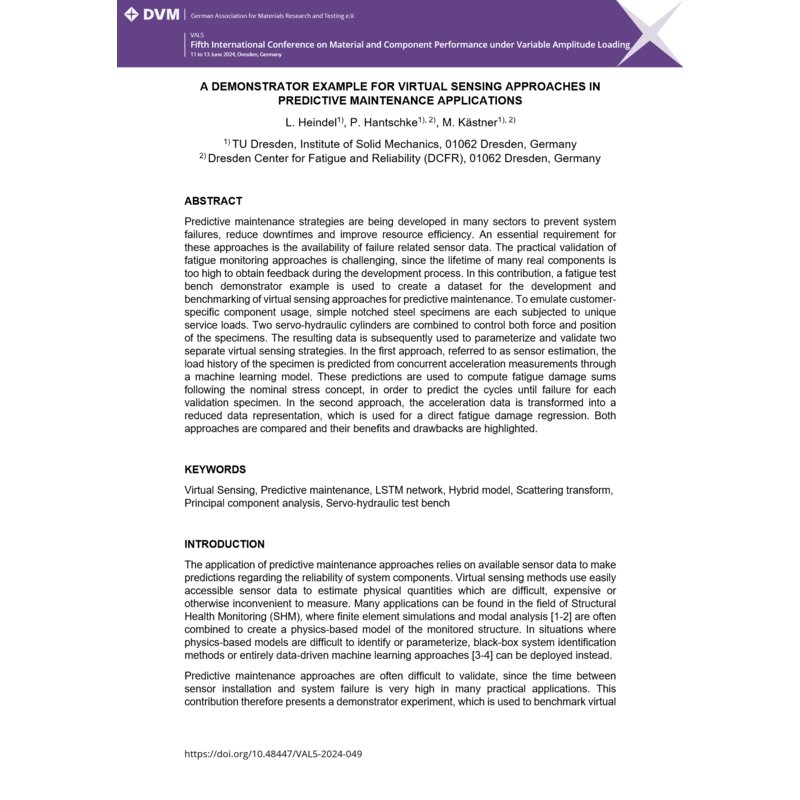
Predictive maintenance strategies are being developed in many sectors to prevent system failures, reduce downtimes and improve resource efficiency. An essential requirement for these approaches is the availability of failure related sensor data at critical components. When monitoring vehicle fleets, acceleration measurements are easily accessible, while extensive sensor setups for each vehicle are not economically feasible. Assuming transferrable system behavior between vehicles, virtual sensing techniques can be deployed to provide estimates for sensor data of interest using predictive data driven models.
The practical validation of predictive maintenance approaches is challenging, since the lifetime of many real components is too high to obtain feedback during the development…
Datenschutzbedingungen (bearbeiten im Modul "Kundenvorteile")
Lieferbedingungen (bearbeiten im Modul "Kundenvorteile")
Rücksendebedingungen (bearbeiten im Modul "Kundenvorteile")
Predictive maintenance strategies are being developed in many sectors to prevent system failures, reduce downtimes and improve resource efficiency. An essential requirement for these approaches is the availability of failure related sensor data at critical components. When monitoring vehicle fleets, acceleration measurements are easily accessible, while extensive sensor setups for each vehicle are not economically feasible. Assuming transferrable system behavior between vehicles, virtual sensing techniques can be deployed to provide estimates for sensor data of interest using predictive data driven models.
The practical validation of predictive maintenance approaches is challenging, since the lifetime of many real components is too high to obtain feedback during the development process. In this contribution, a fatigue test bench demonstrator example is used to create a dataset for the development and benchmarking of virtual sensing approaches for predictive maintenance. To emulate individual user behavior, simple notched steel specimens are each subjected to unique service loads, which are derived from an existing dataset of e-bike measurements [1]. Two servo hydraulic cylinders are combined to control both force and position of the specimens in order to emulate measuring conditions in vehicles.
The resulting data is subsequently used to parameterize and validate two separate virtual sensing strategies. In the first approach [2], referred to as sensor estimation, the load history of the specimen is predicted from concurrent acceleration measurements through a machine learning model. These predictions are used to compute fatigue damage sums following the nominal stress concept, in order to predict the cycles until failure for each validation specimen. In the second approach [3], the acceleration data is transformed into a reduced data representation, which is used to directly predict fatigue damage sums. Both approaches are compared and their benefits and drawbacks are discussed.