- Nur online erhältlich
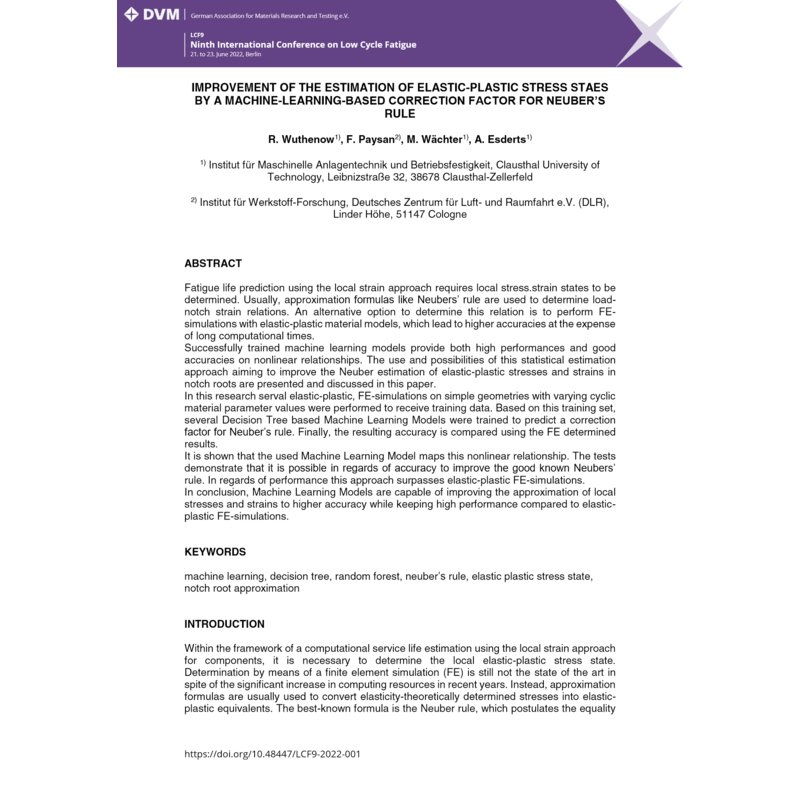
When predicting fatigue life using the notch strain approach local stresses and strains need to be determined. Usually approximation formulas like Neubers’ rule, which are in terms of performance almost unbeatable, are used to determine load-notch strain relations. A second way to determine this relation is to perform FE-simulations with elastic-plastic material models, which lead to higher accuracies at the expense of long calculation times.
Artificial neural networks provide high performance and good accuracy on nonlinear relationships when successfully trained. The use and possibilities of this approach to improve the Neuber estimation of elastic-plastic stresses and strains in notch roots are presented and discussed in this paper.
In this research serval…
Datenschutzbedingungen (bearbeiten im Modul "Kundenvorteile")
Lieferbedingungen (bearbeiten im Modul "Kundenvorteile")
Rücksendebedingungen (bearbeiten im Modul "Kundenvorteile")
When predicting fatigue life using the notch strain approach local stresses and strains need to be determined. Usually approximation formulas like Neubers’ rule, which are in terms of performance almost unbeatable, are used to determine load-notch strain relations. A second way to determine this relation is to perform FE-simulations with elastic-plastic material models, which lead to higher accuracies at the expense of long calculation times.
Artificial neural networks provide high performance and good accuracy on nonlinear relationships when successfully trained. The use and possibilities of this approach to improve the Neuber estimation of elastic-plastic stresses and strains in notch roots are presented and discussed in this paper.
In this research serval elastic-plastic, FE-simulations on simple geometries with varying cyclic material parameter values were performed to get training data with highest accuracy. Using this data, several feed-forward networks with varying hidden unit count and hidden layer count were trained and used to predict the local stress of more complex geometries. The resulting accuracy is compared to analytical approximation formulas and to the FE determined results.
The research finding confirm that artificial neural networks can map this nonlinear relationship. The tests on complex geometries show that it is possible in regards of accuracy to improve the good known Neubers’ rule. In regards of performance, it is shown that this approach surpasses the elastic-plastic FE-simulations.
In conclusion, artificial neural networks are capable of improving the approximation of local stresses and strains to higher accuracy while keeping high performance compared to elastic-plastic FE-simulations.